--------------------------------------
From looking at Dr. Mary (Missy) Louise Cummings her primary background is human/user to machine interface design (jet pilot to control panel, etc), aerial autonomous drones, and some AI, BUT she is not a full-time researcher on FSD or for autonomous ground vehicles on city streets. I am sure she knows much more than the average person about these but she is definitely NOT a world expert on either FSD or AI neural networks for autonomous ground vehicles on city streets.
Dr. Mary Cummings should be opposed not only for bias (besides working for LIDAR company, she also works for Jeff Bezos' Amazon Robotics) she has been proven wrong just like flat-earthers and she refuses to update her views despite clear evidence she is wrong.
Dr. Missy Cummings claimed FSD would require LIDAR/RADAR and that FSD could not be done using cameras only. (see video in next section below)
--------------------------------------
time point: 8:55
Mary "Missy" Cummings says
1. Tesla dropping radar will cause FSD to kill people
2. No researcher believe in camera vision only
Ep.13 Missy Cummings asks: should the US Military use AI weapons?
Jul 12, 2021
The Robot Brains Podcast
Mary "Missy" Cummings is currently a Professor in the Duke University Pratt School of Engineering, the Duke Institute of Brain Sciences, and is the director of the Humans and Autonomy Laboratory and Duke Robotics. Her research interests include human-unmanned vehicle interaction, human-autonomous system collaboration, human-systems engineering, public policy implications of unmanned vehicles, and the ethical and social impact of technology.
--------------------------------------
1. Humans drive using passive optics only (eyes, biologic cameras)
2. Many human drivers with usable vision of only one eye are still permitted & able to drive passenger cars & even commercial trucks.
3. Everyday tens of thousands of mid teen (15-17) drivers take their first drives with safety drivers who are not professionals (their parents or other family) and where the safety drivers have no secondary controls (steering or brakes).
4. Dr. Mary "Missy" Cummings claims Elon is only person that believes camera only. That is false.
Elon & Tesla is not only company that says doing FSD using cameras only is possible.
Outside of Tesla there are other FSD researchers & professionals that believe camera only was possible over 4 years ago, this is many years before Elon & Tesla PROVED it was possible.
5. Elon, Tesla, MobileEye and others have demonstrated camera only is possibly.
Dr. Mary "Missy" Cummings has attitude that FSD systems must initially be perfect even though hundreds of thousands of human drivers cause crashes everyday.
Every year worldwide, human drivers cause accidents resulting in 1.36 million deaths & tens of millions of injuries.
In 2019 USA there were about 35,000 deaths, over 190,000 incapacitating injuries, and over 2 million other injuries.
--------------------------------------
Can You Drive with One Eye?
www.healthline.com
People with monocular vision can legally drive in all 50 U.S. states and in the District of Columbia. If you lose vision in one eye as an adult, you may benefit from visual training activities with an occupational therapist. Learning or relearning to drive with monocular vision is possible. ... Driving with monocular vision.1 mar. 2021
People who grow up with vision in one eye can often judge distance and depth almost as well as a person with vision in both eyes.
If you lose vision in one eye as an adult you, may find it harder to drive, especially at first. If so, you may benefit from working with an occupational therapist or a vision rehabilitation therapist.
With training and practice, many people find that driving and parking are possible and safe.
--------------------------------------
Watch Mobileye’s self-driving car drive through Jerusalem using only cameras
12 cameras, and that’s it
By Andrew J. Hawkins@andyjayhawk Jan 7, 2020
www.theverge.com
--------------------------------------
Predicting the Future from Monocular Cameras in Bird’s-Eye View
22nd April 2021
wayve.ai
.....
We present FIERY: a future instance prediction model in bird’s-eye view from monocular cameras only. Our model predicts future instance segmentation and motion of dynamic agents that can be transformed into non-parametric future trajectories.
Our approach combines the perception, sensor fusion and prediction components of a traditional autonomous driving stack end-to-end, by estimating bird’s-eye-view prediction directly from surround RGB monocular camera inputs. We favour an end-to-end approach as it allows us to directly optimise our representation, rather than decoupling those modules in a multi-stage discrete pipeline of tasks which is prone to cascading errors and high-latency.
Further, classical autonomous driving stacks tackle future prediction by extrapolating the current behaviour of dynamic agents, without taking into account possible interactions. They rely on HD maps and use road connectivity to generate a set of future trajectories. In contrast, FIERY learns to predict future motion of dynamic agents directly from camera driving data in an end-to-end manner, without relying on HD maps or LiDAR sensing. It can reason about the inherent stochastic nature of the future, and predicts multimodal future trajectories
--------------------------------------
Self-driving cars: Lower-cost navigation system - camera-based system
Date January 14, 2015
Contact: [email protected]
.....
The camera-based system still faces many of the same hurdles as laser-based navigation, including how to adapt to varying weather conditions and light levels, as well as unexpected changes in the road. But it’s a valuable new tool in the still-evolving arsenal of technology that’s moving driver-less cars toward reality.
--------------------------------------
Unmanned ground vehicle perception using thermal infrared cameras
May 2011 Proceedings of SPIE - The International Society for Optical Engineering 8045
DOI:10.1117/12.884349
Authors:
Arturo Rankin
Andres Huertas - California Institute of Technology
Larry Matthies
Max Bajracharya
.....
The ability to perform off-road autonomous navigation at any time of day or night is a requirement for some unmanned ground vehicle (UGV) programs. Because there are times when it is desirable for military UGVs to operate without emitting strong, detectable electromagnetic signals, a passive only terrain perception mode of operation is also often a requirement. Thermal infrared (TIR) cameras can be used to provide day and night passive terrain perception.
--------------------------------------
.....
Snuffy • 2017
This is exactly right, I do believe it is possible for camera only system to match and exceed human levels of performance. But we can get their much quicker, by adding extra sensors.
Samcrut • 2017
Babies learn to interpret distance by using their eyes and hands. After a while, we learn how to use just our eyes to gauge distance. Put on a strong pair of glasses and look at how fast your hands go up to re-calibrate your distance measurements. I figure that using LIDAR, RADAR, and SONAR help to accelerate learning, but once the visual systems are suitably calibrated to understand distances based on cameras only, then reliance on other sensors will probably be pulled back. Other sensors might be taken off of the production lines.
--------------------------------------
Leading Causes of Nonfatal Injury Reports, 2000 - 2019, USA
Content source: Centers for Disease Control and Prevention, National Center for Injury Prevention and Control
www.cdc.gov
.....
Some Causes of Nonfatal Unintentional Emergency Department Visits
2019, All Races , Both Sexes , Disposition: All Cases, Ages: 0-85
Cause of Injury Estimated #
Unintentional MV-Occupant 2,107,751
Unintentional Pedal Cyclist 328,094
Unintentional Motorcyclist 206,981
Unintentional Pedestrian 172,605
Definitions Surveillance for Fatal and Nonfatal Injuries --- United States, 2001
Transportation-related:
--- MV-traffic occupant. Injury to a driver or passenger of a motor vehicle caused by a collision, rollover, crash, or other event involving another vehicle, an object, or a pedestrian and occurring on a public highway, street, or road (i.e., originating on, terminating on, or involving a vehicle partially on the highway). This category includes occupants of cars, pickup trucks, vans, heavy transport vehicles, buses, and sport utility vehicles (SUVs). Injuries to occupants of other types of vehicles (e.g., all-terrain vehicles [ATVs], snowmobiles, and go-carts) fall in the other transport category.
--- Motorcyclist: Injury to a driver or passenger of a motorcycle resulting from a collision, loss of control, crash, or other event involving a vehicle, object, or pedestrian. This category includes drivers or passengers of motorcycles (i.e., classic style), sidecars, mopeds, motorized bicycles, and motor-powered scooters.
--- Other transport: Injury to a person boarding, alighting, or riding in or on all other transport vehicles involved in a collision or other event with another vehicle, pedestrian, or animal not described previously. This category includes incidents involving nontraffic or off-road MV collisions, water, air, space, animal, and animal-drawn conveyances (e.g., horseback riding), ATVs, battery-powered carts, ski lifts, and other cable cars not on rails.
--- Pedal cyclist: Injury to a pedal cycle rider from a collision, loss of control, crash, or an event involving a moving vehicle or pedestrian. This category includes riders of unicycles, bicycles, tricycles, and mountain bikes, but excludes injuries unrelated to transportation (i.e., moving) (e.g., repairing a bicycle).
--- Pedestrian (struck by/against a vehicle): Injury to a person involved in a collision, where the person was not at the time of the collision riding in or on an MV, railway train, motorcycle, bicycle, airplane, streetcar, animal-drawn vehicle, or other vehicle. This category includes persons struck by cars, pickup trucks, vans, heavy transport vehicles, buses, and SUVs. This category does not include persons struck by other vehicles (e.g., motorcycles, trains, or bicycles), which are in the other transport category.
--------------------------------------
NHTSA Traffic Safety Facts Annual Report Tables
--------------------------------------
In the crash causal chain, driver was assigned 94 percent (±2.2%) of the time
Critical Reasons for Crashes Investigated in the
National Motor Vehicle Crash Causation Survey
Published by NHTSA’s National Center for Statistics and Analysis
1200 New Jersey Avenue SE., Washington, DC 20590
TRAFFIC SAFETY FACTS Crash
StatsDOT HS 812 115 A Brief Statistical Summary
February 2015
SummaryThe National Motor Vehicle Crash Causation Survey (NMVCCS), conducted from 2005 to 2007, was aimed at collecting on-scene information about the events and associated factors leading up to crashes involving light vehicles. Several facets of crash occurrence were investigated during data collection, namely the pre-crash movement, critical pre-crash event, critical reason, and the associated factors. A weighted sample of 5,470 crashes was investigated over a period of two and a half years, which represents an estimated 2,189,000 crashes nationwide. About 4,031,000 vehicles, 3,945,000 drivers, and 1,982,000 passengers were estimated to have been involved in these crashes. The critical reason, which is the last event in the crash causal chain, was assigned to the driver in 94 percent (±2.2%)† of the crashes. In about 2 percent (±0.7%) of the crashes, the critical reason was assigned to a vehicle component’s
failure or degradation, and in 2 percent (±1.3%) of crashes, it was attributed to the environment (slick roads, weather, etc.). Among an estimated 2,046,000 drivers who were assigned critical reasons, recognition errors accounted for about 41 percent (±2.1%), decision errors 33 percent (±3.7%), and performance errors 11 percent (±2.7%) of the crashes.
.....
Driver‑Related Critical Reasons
Critical reason attributed to drivers
The critical reason was assigned to drivers in an estimated 2,046,000
crashes that comprise 94 percent of the NMVCCS crashes at the
national level. However, in none of these cases was the assignment
intended to blame the driver for causing the crash. The driver-
related critical reasons are broadly classified into recognition
errors, decision errors, performance errors, and non- performance
errors. Statistics in Table 2 show that the recognition error, which
included driver’s inattention, internal and external distractions,
and inadequate surveillance, was the most (41% ±2.2%) frequently
assigned critical reason. Decision error such as driving too fast
for conditions, too fast for the curve, false assumption of others’
actions, illegal maneuver and misjudgment of gap or others’ speed
accounted for about 33 percent (±3.7%) of the crashes. In about 11
percent (±2.7%) of the crashes, the critical reason was performance
error such as overcompensation, poor directional control, etc.
Sleep was the most common critical reason among non-perfor-
mance errors that accounted for 7 percent (±1.0%) of the crashes.
Other driver errors were recorded as critical reasons for about 8
percent (±1.9%) of the drivers.
--------------------------------------
THE RELATIVE FREQUENCY OF UNSAFE DRIVING ACTS IN SERIOUS TRAFFIC CRASHES
THE RELATIVE FREQUENCY OF UNSAFE DRIVING ACTS IN SERIOUS TRAFFIC CRASHES
SUMMARY OF IMPORTANT FINDINGS
This study was conducted to determine the specific driver behaviors and unsafe driving acts (UDAs) that lead to crashes, and the situational, driver and vehicle characteristics associated with these behaviors. A sample of 723 crashes involving 1284 drivers was investigated at four different sites in the country during the period from April 1, 1996 through April 30, 1997. The crashes were selected using the National Automotive Sampling System (NASS) protocol and provided a fair sample of serious crashes involving passenger vehicles in the United States. In-depth data were collected and evaluated on the condition of the vehicles, the crash scene, roadway conditions, driver behaviors and situational factors at the time of the crash. Investigators used an 11 step process to evaluate the crash, determine the primary cause of each crash, and uncover contributing factors.
Crash causes were attributed to either driver behavior or other causes. In 717 of the 723 crashes investigated (99%), a driver behavioral error caused or contributed to the crash. Of the 1284 drivers involved in these crashes, 732 drivers (57%) contributed in some way to the cause of their crashes. There were six causal factors associated with driver behaviors that occurred at relatively high frequencies for these drivers and accounted for most of the problem behaviors. They were:
DRIVER INATTENTION 22.7%
VEHICLE SPEED 18.7%
ALCOHOL IMPAIRMENT 18.2%
PERCEPTUAL ERRORS (e.g. looked, but didn't see) 15.1%
DECISION ERRORS (e.g. turned with obstructed view) 10.1%
INCAPACITATION (e.g. fell asleep) 6.4%
Problem types in terms of crash configuration and specific behavioral errors were also identified. The following seven crash problem types, when associated with specific behavioral errors, accounted for almost half of the crashes studied where there was a driver behavioral error:
SAME DIRECTION, REAR END - (Driver Inattention Factors) 12.9%
TURN, MERGE, PATH ENCROACHMENT- (Looked, Did Not See, etc.) 12.0%
SINGLE DRIVER, ROADSIDE DEPARTURE - (Speed, Alcohol) 10.3%
INTERSECTING PATHS, STRAIGHT PATHS - (Looked, Did Not See, etc.) 4.1%
SAME TRAFFIC-WAY, OPPOSITE DIRECTION - (Inattention, Speed) 2.6%
BACKING, OTHER, MISCELLANEOUS, ETC. - (Following Too Closely, Speed) 1.3%
--------------------------------------
From looking at Dr. Mary (Missy) Louise Cummings her primary background is human/user to machine interface design (jet pilot to control panel, etc), aerial autonomous drones, and some AI, BUT she is not a full-time researcher on FSD or for autonomous ground vehicles on city streets. I am sure she knows much more than the average person about these but she is definitely NOT a world expert on either FSD or AI neural networks for autonomous ground vehicles on city streets.
Dr. Mary Cummings should be opposed not only for bias (besides working for LIDAR company, she also works for Jeff Bezos' Amazon Robotics) she has been proven wrong just like flat-earthers and she refuses to update her views despite clear evidence she is wrong.
Dr. Missy Cummings claimed FSD would require LIDAR/RADAR and that FSD could not be done using cameras only. (see video in next section below)
--------------------------------------
time point: 8:55
Mary "Missy" Cummings says
1. Tesla dropping radar will cause FSD to kill people
2. No researcher believe in camera vision only
Ep.13 Missy Cummings asks: should the US Military use AI weapons?
Jul 12, 2021
The Robot Brains Podcast
Mary "Missy" Cummings is currently a Professor in the Duke University Pratt School of Engineering, the Duke Institute of Brain Sciences, and is the director of the Humans and Autonomy Laboratory and Duke Robotics. Her research interests include human-unmanned vehicle interaction, human-autonomous system collaboration, human-systems engineering, public policy implications of unmanned vehicles, and the ethical and social impact of technology.

--------------------------------------
1. Humans drive using passive optics only (eyes, biologic cameras)
2. Many human drivers with usable vision of only one eye are still permitted & able to drive passenger cars & even commercial trucks.
3. Everyday tens of thousands of mid teen (15-17) drivers take their first drives with safety drivers who are not professionals (their parents or other family) and where the safety drivers have no secondary controls (steering or brakes).
4. Dr. Mary "Missy" Cummings claims Elon is only person that believes camera only. That is false.
Elon & Tesla is not only company that says doing FSD using cameras only is possible.
Outside of Tesla there are other FSD researchers & professionals that believe camera only was possible over 4 years ago, this is many years before Elon & Tesla PROVED it was possible.
5. Elon, Tesla, MobileEye and others have demonstrated camera only is possibly.
Dr. Mary "Missy" Cummings has attitude that FSD systems must initially be perfect even though hundreds of thousands of human drivers cause crashes everyday.
Every year worldwide, human drivers cause accidents resulting in 1.36 million deaths & tens of millions of injuries.
In 2019 USA there were about 35,000 deaths, over 190,000 incapacitating injuries, and over 2 million other injuries.
--------------------------------------
Can You Drive with One Eye?
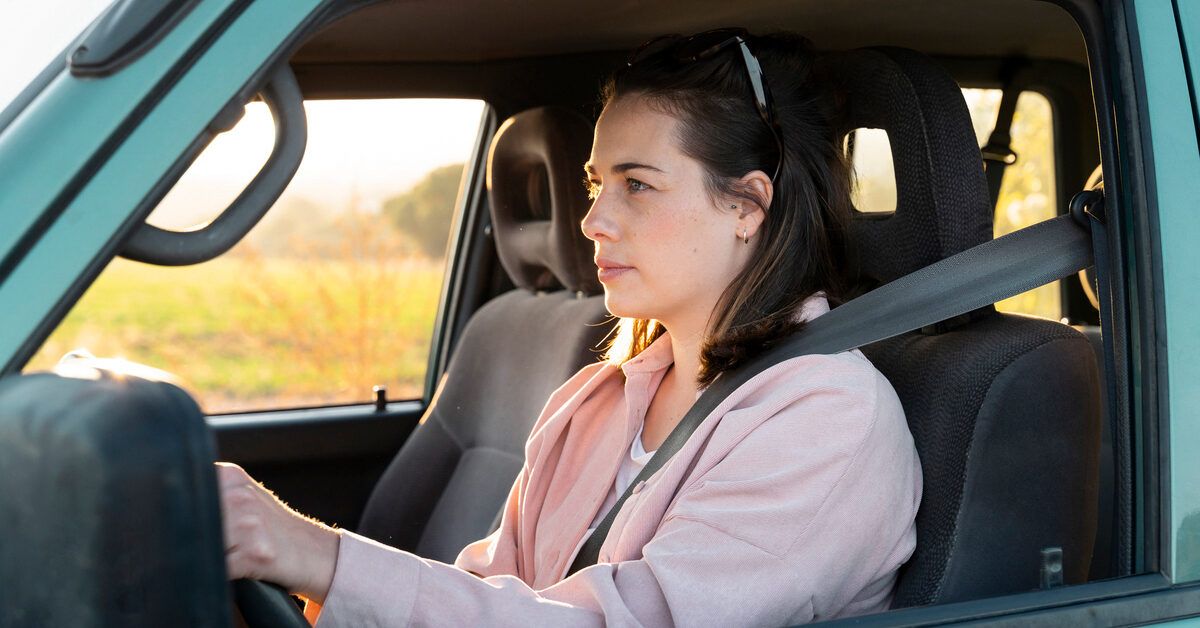
Can You Drive with One Eye? Limitations and Safety
Driving with vision in one eye is possible. We’ll talk about any limitations you might experience.
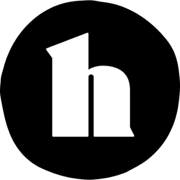
People with monocular vision can legally drive in all 50 U.S. states and in the District of Columbia. If you lose vision in one eye as an adult, you may benefit from visual training activities with an occupational therapist. Learning or relearning to drive with monocular vision is possible. ... Driving with monocular vision.1 mar. 2021
People who grow up with vision in one eye can often judge distance and depth almost as well as a person with vision in both eyes.
If you lose vision in one eye as an adult you, may find it harder to drive, especially at first. If so, you may benefit from working with an occupational therapist or a vision rehabilitation therapist.
With training and practice, many people find that driving and parking are possible and safe.
--------------------------------------
Watch Mobileye’s self-driving car drive through Jerusalem using only cameras
12 cameras, and that’s it
By Andrew J. Hawkins@andyjayhawk Jan 7, 2020
/cdn.vox-cdn.com/uploads/chorus_asset/file/19585404/mobileeye_grab.jpg)
Watch Mobileye’s self-driving car drive through Jerusalem using only cameras
The car navigates complex driving situations.

--------------------------------------
Predicting the Future from Monocular Cameras in Bird’s-Eye View
22nd April 2021
Predicting the future from monocular cameras in bird’s-eye view
Driving can often result in situations where the immediate decision is not obvious if you only take a single snapshot of the world

.....
We present FIERY: a future instance prediction model in bird’s-eye view from monocular cameras only. Our model predicts future instance segmentation and motion of dynamic agents that can be transformed into non-parametric future trajectories.
Our approach combines the perception, sensor fusion and prediction components of a traditional autonomous driving stack end-to-end, by estimating bird’s-eye-view prediction directly from surround RGB monocular camera inputs. We favour an end-to-end approach as it allows us to directly optimise our representation, rather than decoupling those modules in a multi-stage discrete pipeline of tasks which is prone to cascading errors and high-latency.
Further, classical autonomous driving stacks tackle future prediction by extrapolating the current behaviour of dynamic agents, without taking into account possible interactions. They rely on HD maps and use road connectivity to generate a set of future trajectories. In contrast, FIERY learns to predict future motion of dynamic agents directly from camera driving data in an end-to-end manner, without relying on HD maps or LiDAR sensing. It can reason about the inherent stochastic nature of the future, and predicts multimodal future trajectories
--------------------------------------
Self-driving cars: Lower-cost navigation system - camera-based system
Date January 14, 2015
Contact: [email protected]

Self-driving cars: Lower-cost navigation system developed
A new software system developed at the University of Michigan uses video game technology to help solve one of the most daunting hurdles facing self-driving and automated cars—the high cost of the laser scanners they use to determine their location.
news.umich.edu
.....
The camera-based system still faces many of the same hurdles as laser-based navigation, including how to adapt to varying weather conditions and light levels, as well as unexpected changes in the road. But it’s a valuable new tool in the still-evolving arsenal of technology that’s moving driver-less cars toward reality.
--------------------------------------
Unmanned ground vehicle perception using thermal infrared cameras
May 2011 Proceedings of SPIE - The International Society for Optical Engineering 8045
DOI:10.1117/12.884349
Authors:
Arturo Rankin
Andres Huertas - California Institute of Technology
Larry Matthies
Max Bajracharya
.....
The ability to perform off-road autonomous navigation at any time of day or night is a requirement for some unmanned ground vehicle (UGV) programs. Because there are times when it is desirable for military UGVs to operate without emitting strong, detectable electromagnetic signals, a passive only terrain perception mode of operation is also often a requirement. Thermal infrared (TIR) cameras can be used to provide day and night passive terrain perception.
--------------------------------------
.....
Snuffy • 2017
This is exactly right, I do believe it is possible for camera only system to match and exceed human levels of performance. But we can get their much quicker, by adding extra sensors.
Samcrut • 2017
Babies learn to interpret distance by using their eyes and hands. After a while, we learn how to use just our eyes to gauge distance. Put on a strong pair of glasses and look at how fast your hands go up to re-calibrate your distance measurements. I figure that using LIDAR, RADAR, and SONAR help to accelerate learning, but once the visual systems are suitably calibrated to understand distances based on cameras only, then reliance on other sensors will probably be pulled back. Other sensors might be taken off of the production lines.
--------------------------------------
Leading Causes of Nonfatal Injury Reports, 2000 - 2019, USA
Content source: Centers for Disease Control and Prevention, National Center for Injury Prevention and Control
Injury Center | CDC
The CDC Injury Center focuses on how injury and violence impact all of us and how to prevent it. Focus areas are overdose, suicide, and adverse childhood experiences (ACEs).

.....
Some Causes of Nonfatal Unintentional Emergency Department Visits
2019, All Races , Both Sexes , Disposition: All Cases, Ages: 0-85
Cause of Injury Estimated #
Unintentional MV-Occupant 2,107,751
Unintentional Pedal Cyclist 328,094
Unintentional Motorcyclist 206,981
Unintentional Pedestrian 172,605
Definitions Surveillance for Fatal and Nonfatal Injuries --- United States, 2001
Transportation-related:
--- MV-traffic occupant. Injury to a driver or passenger of a motor vehicle caused by a collision, rollover, crash, or other event involving another vehicle, an object, or a pedestrian and occurring on a public highway, street, or road (i.e., originating on, terminating on, or involving a vehicle partially on the highway). This category includes occupants of cars, pickup trucks, vans, heavy transport vehicles, buses, and sport utility vehicles (SUVs). Injuries to occupants of other types of vehicles (e.g., all-terrain vehicles [ATVs], snowmobiles, and go-carts) fall in the other transport category.
--- Motorcyclist: Injury to a driver or passenger of a motorcycle resulting from a collision, loss of control, crash, or other event involving a vehicle, object, or pedestrian. This category includes drivers or passengers of motorcycles (i.e., classic style), sidecars, mopeds, motorized bicycles, and motor-powered scooters.
--- Other transport: Injury to a person boarding, alighting, or riding in or on all other transport vehicles involved in a collision or other event with another vehicle, pedestrian, or animal not described previously. This category includes incidents involving nontraffic or off-road MV collisions, water, air, space, animal, and animal-drawn conveyances (e.g., horseback riding), ATVs, battery-powered carts, ski lifts, and other cable cars not on rails.
--- Pedal cyclist: Injury to a pedal cycle rider from a collision, loss of control, crash, or an event involving a moving vehicle or pedestrian. This category includes riders of unicycles, bicycles, tricycles, and mountain bikes, but excludes injuries unrelated to transportation (i.e., moving) (e.g., repairing a bicycle).
--- Pedestrian (struck by/against a vehicle): Injury to a person involved in a collision, where the person was not at the time of the collision riding in or on an MV, railway train, motorcycle, bicycle, airplane, streetcar, animal-drawn vehicle, or other vehicle. This category includes persons struck by cars, pickup trucks, vans, heavy transport vehicles, buses, and SUVs. This category does not include persons struck by other vehicles (e.g., motorcycles, trains, or bicycles), which are in the other transport category.
--------------------------------------
NHTSA Traffic Safety Facts Annual Report Tables
--------------------------------------
In the crash causal chain, driver was assigned 94 percent (±2.2%) of the time
Critical Reasons for Crashes Investigated in the
National Motor Vehicle Crash Causation Survey
Published by NHTSA’s National Center for Statistics and Analysis
1200 New Jersey Avenue SE., Washington, DC 20590
TRAFFIC SAFETY FACTS Crash
StatsDOT HS 812 115 A Brief Statistical Summary
February 2015
SummaryThe National Motor Vehicle Crash Causation Survey (NMVCCS), conducted from 2005 to 2007, was aimed at collecting on-scene information about the events and associated factors leading up to crashes involving light vehicles. Several facets of crash occurrence were investigated during data collection, namely the pre-crash movement, critical pre-crash event, critical reason, and the associated factors. A weighted sample of 5,470 crashes was investigated over a period of two and a half years, which represents an estimated 2,189,000 crashes nationwide. About 4,031,000 vehicles, 3,945,000 drivers, and 1,982,000 passengers were estimated to have been involved in these crashes. The critical reason, which is the last event in the crash causal chain, was assigned to the driver in 94 percent (±2.2%)† of the crashes. In about 2 percent (±0.7%) of the crashes, the critical reason was assigned to a vehicle component’s
failure or degradation, and in 2 percent (±1.3%) of crashes, it was attributed to the environment (slick roads, weather, etc.). Among an estimated 2,046,000 drivers who were assigned critical reasons, recognition errors accounted for about 41 percent (±2.1%), decision errors 33 percent (±3.7%), and performance errors 11 percent (±2.7%) of the crashes.
.....
Driver‑Related Critical Reasons
Critical reason attributed to drivers
The critical reason was assigned to drivers in an estimated 2,046,000
crashes that comprise 94 percent of the NMVCCS crashes at the
national level. However, in none of these cases was the assignment
intended to blame the driver for causing the crash. The driver-
related critical reasons are broadly classified into recognition
errors, decision errors, performance errors, and non- performance
errors. Statistics in Table 2 show that the recognition error, which
included driver’s inattention, internal and external distractions,
and inadequate surveillance, was the most (41% ±2.2%) frequently
assigned critical reason. Decision error such as driving too fast
for conditions, too fast for the curve, false assumption of others’
actions, illegal maneuver and misjudgment of gap or others’ speed
accounted for about 33 percent (±3.7%) of the crashes. In about 11
percent (±2.7%) of the crashes, the critical reason was performance
error such as overcompensation, poor directional control, etc.
Sleep was the most common critical reason among non-perfor-
mance errors that accounted for 7 percent (±1.0%) of the crashes.
Other driver errors were recorded as critical reasons for about 8
percent (±1.9%) of the drivers.
--------------------------------------
THE RELATIVE FREQUENCY OF UNSAFE DRIVING ACTS IN SERIOUS TRAFFIC CRASHES

THE RELATIVE FREQUENCY OF UNSAFE DRIVING ACTS IN SERIOUS TRAFFIC CRASHES
SUMMARY OF IMPORTANT FINDINGS
This study was conducted to determine the specific driver behaviors and unsafe driving acts (UDAs) that lead to crashes, and the situational, driver and vehicle characteristics associated with these behaviors. A sample of 723 crashes involving 1284 drivers was investigated at four different sites in the country during the period from April 1, 1996 through April 30, 1997. The crashes were selected using the National Automotive Sampling System (NASS) protocol and provided a fair sample of serious crashes involving passenger vehicles in the United States. In-depth data were collected and evaluated on the condition of the vehicles, the crash scene, roadway conditions, driver behaviors and situational factors at the time of the crash. Investigators used an 11 step process to evaluate the crash, determine the primary cause of each crash, and uncover contributing factors.
Crash causes were attributed to either driver behavior or other causes. In 717 of the 723 crashes investigated (99%), a driver behavioral error caused or contributed to the crash. Of the 1284 drivers involved in these crashes, 732 drivers (57%) contributed in some way to the cause of their crashes. There were six causal factors associated with driver behaviors that occurred at relatively high frequencies for these drivers and accounted for most of the problem behaviors. They were:
DRIVER INATTENTION 22.7%
VEHICLE SPEED 18.7%
ALCOHOL IMPAIRMENT 18.2%
PERCEPTUAL ERRORS (e.g. looked, but didn't see) 15.1%
DECISION ERRORS (e.g. turned with obstructed view) 10.1%
INCAPACITATION (e.g. fell asleep) 6.4%
Problem types in terms of crash configuration and specific behavioral errors were also identified. The following seven crash problem types, when associated with specific behavioral errors, accounted for almost half of the crashes studied where there was a driver behavioral error:
SAME DIRECTION, REAR END - (Driver Inattention Factors) 12.9%
TURN, MERGE, PATH ENCROACHMENT- (Looked, Did Not See, etc.) 12.0%
SINGLE DRIVER, ROADSIDE DEPARTURE - (Speed, Alcohol) 10.3%
INTERSECTING PATHS, STRAIGHT PATHS - (Looked, Did Not See, etc.) 4.1%
SAME TRAFFIC-WAY, OPPOSITE DIRECTION - (Inattention, Speed) 2.6%
BACKING, OTHER, MISCELLANEOUS, ETC. - (Following Too Closely, Speed) 1.3%
--------------------------------------